-
Statutory audits
As a member firm of Grant Thornton International (GTIL), we provide international-standard audit & assurance services. We perform audit services effectively and efficiently by utilising our global audit methodologyo deliver consistent audit services globally and the integrated audit tool (Leap) to maintain audit quality and improve audit efficiency. Also our audit services are regularly reviewed by GTIL review team, thereby allowing us to further improve our audit quality.
-
IPO voluntary audits
Grant Thornton Taiyo LLC provides audits for preparing for public offering, based on its extensive experience, to many companies aiming to go public.
-
Other voluntary audits
Grant Thornton Taiyo LLC provides various types of discretionary audits based on the request of management.
-
Information security audit
We verify and evaluate the state of appropriate controls based on information security risk assessments, and provide accurate advice to help improve information security.
-
Business Tax Services
Growing businesses need strong tax management to meet current and future tax liabilities and we can help you achieve this, whatever challenges you face.
-
Private Client Consulting
Private Client Consulting team provides a comprehensive cross section of advisory services to high net worth individuals and corporate executives, allowing such individuals to concentrate on their business interests.
-
International Tax Services
In an ever-increasing environment of global competition, businesses must now cope with not only the complexity of different global operating environments, but also with global tax, legislative and corporate regulatory requirements.
-
Transaction Tax
Our collaborative approach creates dynamic teams with a range of financial, commercial and operational backgrounds. We can help you understand the value drivers behind successful transactions. We support you throughout the transaction process helping achieve the best possible outcome at the point of the transaction and in the longer term.
-
Human Capital
With expertise in visa, payroll, human capital and income tax, we adopt a multi-disciplinary and global approach to provide comprehensive solutions to businesses' most valuable asset-people.
-
Corporate Finance
Growing your business profitably against a tough economic backdrop is challenging and correctly evaluating and delivering transactions is a key part of a company’s growth strategy.
-
IPO Consulting
From business incubation to public stock offering, we offer a wide range of support programsservices for every stage of business development.
-
International financial reporting standards (IFRS)
Our IFRS advisers can help you navigate the complexity of the Standards so you can focus your time and effort on running your business.
-
Reorganizations
Grant Thornton Taiyo Inc. provides total support for corporate restructuring; aimed atto responding to dramatic changes in the management environment.
-
Corporate governance and risk management
Helping you balance risk and opportunity
-
Forensic and investigation services
Rapid and customised approach to investigations and dispute resolution
-
Market research
Growing your business profitably against a tough economic backdrop is challenging and correctly evaluating and delivering transactions is a key part of a company’s growth strategy.
-
Strategy
We can support you throughout the transaction process – helping achieve the best possible outcome at the point of the transaction and in the longer term..
-
China Business and Tax Advisory Services
We take the worry out of doing business in China, working closely with Grant Thornton member firms and other professional accounting firms in China.
-
Business Process Re-engineering
Growing your business profitably against a tough economic backdrop is challenging and correctly evaluating and delivering transactions is a key part of a company’s growth strategy.
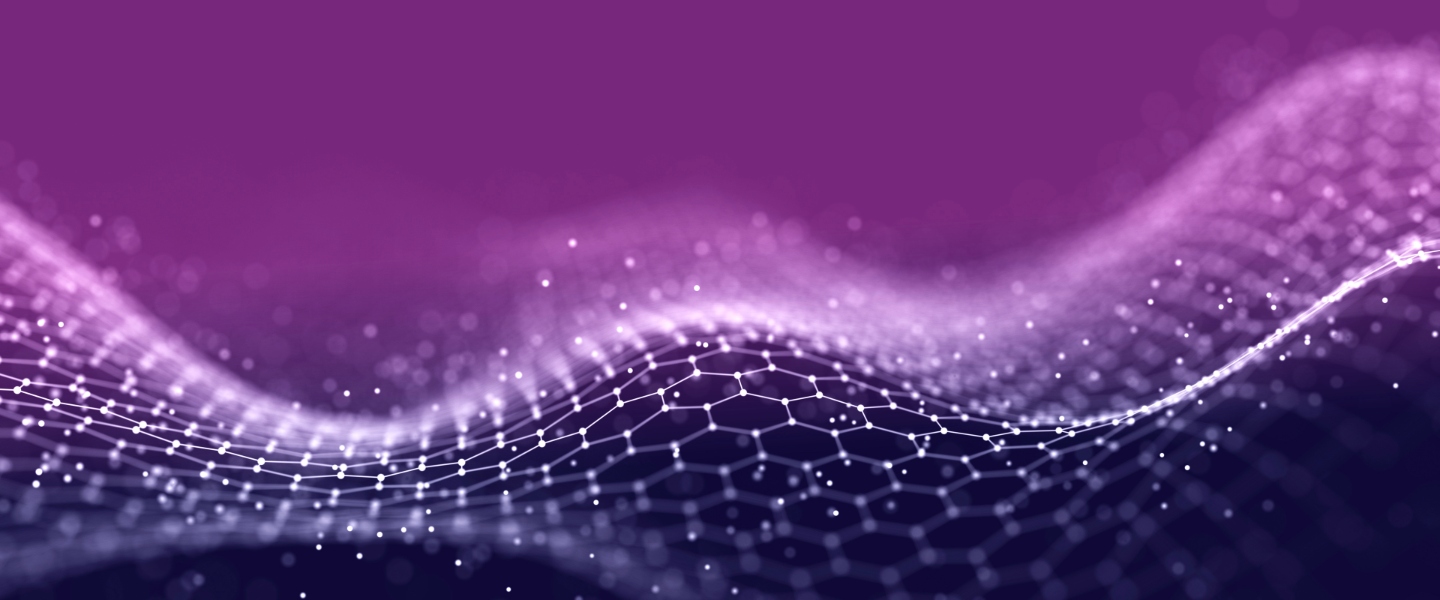
View as PDF
The quality of AI outputs is improving with a pace too significant for it to be discounted. The oft repeated phrase is that AI and automation is ideal for tasks that are repetitive, follow a specific set of rules, and take a long period of time with manual input. For example, current AI can assimilate information from a Local File and use this to evaluate the comparability of comparable companies based on their business description and other information, providing acceptance and rejection reasons accordingly.
When it comes to transfer pricing, the TNMM (Transaction Net Margin Method) benchmarking studies are an ideal task for AI to tackle, and there are emerging TP specialized AI systems, as well as generalized AI such as ChatGPT that already exist to select companies with comparable functions and risks, thus forming a benchmarked range of profit margins to evaluate the arm’s length nature of intercompany transactions.
However, the use of AI is not yet widely adopted, and there remain concerns over how accurately AI can consistently judge comparability without being fooled by “marketing speak” from company information on websites and annual reports, as well as potentially unclear and occasion inaccurate information on commercial databases widely used by TP practitioners. If AI can perform judgements quickly and accurately, questions relating to the human professional’s role, and how tax authorities may utilize AI will inevitably arise. Furthermore, all Japanese listed companies have extensive disclosed corporate overview information and financial information systematically listed in the company annual reports due to the financial services agency requiring high quality corporate disclosures available online. This information is generally only available in Japanese, representing a language barrier for the human professional, which can be overcome by AI. This is also the case for annual reports disclosed in other markets, but the fact that Japan company annual reports always have the same specific information items disclosed (further explained in chapter 3 of this article), means that the qualitative analysis of comparables can be particularly easily standardized in the Japanese market. This is unlike disclosures in other markets where information provided can vary from company to company.
1. AI and qualitative analysis
When selecting comparable companies, it is not necessarily a simple choice of accepting companies that seem largely similar and rejecting those that deal with dissimilar functions or products. Depending on the industry, there may be few companies that are directly comparable, and a loosening of comparability standards for products or functions may be necessary. For example, with a confectionary distribution company, a drinks distribution company could be considered to have comparable functions and risks, but an alcohol distribution company could be argued to be less comparable, given the particular risks and licensing requirements related to alcohol. Further, a benchmarked set should ensure that it supports the taxpayer profit results and pricing policy, but should also be robust and defensible to tax authority scrutiny.
A benchmarked set that only chooses companies that are almost identical to the tested party, but which do not support the tested party pricing or profit results are of little value, and nor is a benchmarked set that supports the tested party profit results but falls apart under tax authority scrutiny. In these cases, the transfer pricing professional must disseminate the relevant comparable company information and make judgments accordingly, with clearly documented logical and consistent rationale for the acceptance or rejection of each company reviewed. This is the task that AI must undertake.
2. Exploiting AI to its potential
In order to perform the above explained judgments, a nuanced understanding of AI prompting is of primordial importance. AI can perform certain arduous and repetitive tasks extremely well when accurately prompted, but can still be prone to “hallucinations” or making judgments that do not take into account certain factors that the human professional may assume as a given to consider. Further, one must consider the information that the AI is disseminating - if company websites are used, these will often provide persuasive messages on how the company provides a full suite of services or products and offers unique value, in order to persuade customers and investors accordingly. However, this may not provide an accurate account of the actual economic characterization of the potential comparable, and any evaluation must collate and process the information with an understanding of what the company actually does. There must therefore be careful consideration on how the AI makes such judgments.
For example, if the tested party is a limited risk distributor of PC products, performing a certain level of local marketing, but primarily following the instructions and strategy of the overseas affiliate, it may be prudent to prompt elimination of a distributor that has any proprietary technology or trademark that may generate a higher level of value add. However, further scrutiny is also required to investigate whether any proprietary assets genuinely represents a significant influence on excess profits or losses. It may be the case that AI can learn these considerations over time and incorporate it in future strategies, but it will be key for human professionals to ensure that AI maps out its rationale clearly for each comparable, tailored to the tested party in question, as well as review each judgment made for consistency and defensibility in a tax audit. One great advantage is of course that if an additional consideration needs to be made during a qualitative analysis, AI can repeat this with all other remaining companies in a fraction of the time, but must once again be reviewed by TP professionals to ensure that judgments made are not only accurate, but practicable for the requirements of the particular situation at hand.
3. Characteristics of Japan market benchmarks and AI's potential role
Such considerations may be particularly easy to transparently perform in benchmarking studies of Japanese listed companies. This is because the company annual reports (Yuka Shoken Hokokusho, or “Yuho” for short) provide the same information in a set format for each company. Therefore, in a Japanese benchmarking study, it is possible to check the following items to inform judgment of comparability. (Not an exhaustive list)
- Sales per segment
One can evaluate whether a certain product or functional segment is dominant or not. In this way, if a non-comparable segment has negligible sales, this can be easily checked and evidenced. Occasionally, some companies do not report segmented profits, and instead report all activities under “one single segment”.
- Transaction reliance
It is necessary for Japanese listed companies to report the level of sales with significant customers. If the majority of sales are to one or two customers, losing those customers will have a significant impact on profit fluctuations, and could constitute a significant risk that renders the company non comparable.
- Manufacturing costs
While the business description of some companies may not explicitly refer to extensive manufacturing, the manufacturing costs may constitute a high proportion of sales. This can be easily checked in a Yuho and form a clear reason for rejection of a company.
- Sales in different geographic markets
The level of sales to markets in and outside Japan must be reported - if the majority of sales are to non-Japanese customers, this could also form a rationale for rejection.
Searching for these figures is a simple, repetitive, time consuming, but important job. Such analysis could easily be performed and output by AI, and potentially lead to a certain level of standardization of review methods with Japanese listed companies. This is particularly true as all Yuho reports are formatted in easily readable text, so the issue of reviewing annual reports with text that may be misread by AI is minimized. This may also be particularly relevant for bilateral and unilateral APA applications which are relatively popular in the Japan market, as this can provide a certain level of clarity and objectivity to the review of any TNMM benchmark utilized in an APA application that may need to be accepted by one or two sets of competent authorities.
Unlike other markets, Japanese transfer pricing reports tend not to use “off the shelf” standard benchmarking studies often, unless part of a larger set of global documentation. This is in part due to the ability to review detailed information in the Yuho report, as well as the tax authority’s view that Japan is a market with unique characteristics, which lends to more tailored benchmarking for each company. However, with the potential of increased efficient systemization of Yuho review by AI, which can also fully document the rationale for acceptance or rejection of a comparable company, it may be more feasible to generate sets of often used benchmarking studies and request AI to verify it against the functional analysis of a similar company, and fine tune accordingly.
An additional factor is that the universes of companies used for Japanese benchmarking searches are generally smaller than those of many other jurisdictions, due to the fact that only Japanese companies are generally accepted by the Japanese tax authorities, eliminating the need to evaluate other APAC comparable companies. Despite this, quantitative screening criteria are still used to filter out companies that may have excessive R&D expenses, or have consistent operating losses. However, some filters such as revenue levels do not always have a fully explainable rationale as to how they affect function/risk comparability and profit margins, and instead may be used mainly to reduce sample size for manual qualitative review. However, with the increased efficiency of AI, this may lead to less use of quantitative screenings with murky rationale, as qualitative review of numerous companies will be far less time consuming with AI, assuming that the appropriate prompts as explained above can be effectively applied. Alternatively, with AI easily being able to calculate multiple different financial ratios from the profit and loss statements or balance sheet, AI may facilitate the use of more rational quantitative screens which could be deployed more efficiently.
Given the potential advances explained above, could the Japan tax authorities use AI in their tax audits, or even to generate sets of “secret comparables” using a black box of prompts? In many jurisdictions, including Japan, the tax authorities have extremely strict confidentiality requirements when receiving company information, and have strict procedures in place to ensure that company information is not leaked. Indeed, the Japan tax authorities generally do not correspond with companies or tax advisers by email for this very reason. Due to this, it will likely be highly challenging for the Japanese tax authorities to directly use AI for benchmarking analyses, directly based on company information, However, it is an undeniable possibility that AI could be used generally by the tax authorities to enhance their benchmarking analysis capacity, and for example generating a set of secret comparables based on general criteria based on their current understanding of the taxpayer’s functions and risks, without having to use company information. Further, if information encryption and security of AI highly advances over the coming years, while the Japan tax authorities may still be hesitant to adopt AI, there may be tax authorities in other jurisdictions that may be more willing to adopt AI-based benchmarking, leading to differences in benchmarking approaches between tax authorities in tax audits or bilateral APA applications. While the use of AI by tax authorities remains to be seen, the possibility prompts taxpayers and advisers to get the first mover advantage on maximizing the use of AI accordingly.
4. Conclusion
The use of AI in benchmarking is inevitable, and like many other fields, TP professionals will have to understand how to maximize the use of AI and develop nuanced promoting skills. In the future, with the current exponential development curve, it is likely that AI will be able to provide an efficient analysis and description of the functions and risks of a tested party, and accurately delineate the comparability of comparable companies, providing consistent rationale for a benchmarking study. Currently, this is reliant on tax professionals providing the correct information and prompts to AI, such as an accurate description of the functional analysis of the tested party, which AI is currently not always able to accurately analyse with 100% accuracy at this time. While AI is likely to replace much of the base-level benchmarking analysis that professionals currently perform, it will be the role of human professionals to direct and tailor the use of AI accordingly. In order to do so, the analysis performed by AI must be underpinned by an understanding of the facts and circumstances of each client business, as well as the context of the analysis (whether the benchmarking study is for a local file, tax assessment or bilateral APA etc.) for which the pragmatic input of the human professional will remain essential.
If you are interested in receiving our latest insights, You can sign up to our mailing list.